This PhD project focuses on using machine learning to improve how we identify and analyse features in the light spectra from galaxies. The goal is to expand the types of spectral templates we use and improve how we process the data to make the analysis faster and more efficient. Most research so far has relied on a single spectral library, but this project will explore other libraries and combinations of them to see how they affect the accuracy of important measurements, such as the age of stars, the amount of metals in them, dust levels, star formation rates, and stellar mass distribution. The overall aim is to make these estimates more precise across different types of stellar populations, helping us better understand how galaxies evolve.
The project will use various machine learning techniques, including neural networks and deep learning models, to see how well they can interpret and model spectral data. One important part of the project will be to improve these models so they can handle different combinations of spectral templates, perform well with noisy data, and make reliable predictions under different conditions. Explainable AI methods will be used to make the machine learning models more transparent and easier to understand. These techniques will help show which parts of the spectral data influence the model’s predictions, making it easier to trust the results, especially with complex or noisy data. This approach will lead to more accurate predictions and allow machine learning to be used more widely in analysing spectral data from a variety of galaxies.
Another key part of the project will be to identify and correct biases that different spectral libraries might introduce. The project will provide both theoretical and practical tools to help astronomers interpret large amounts of spectral data with greater accuracy, efficiency, and clarity.
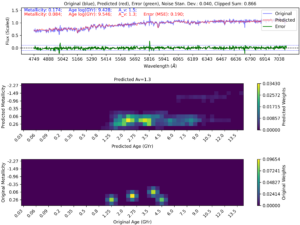